GET READY TO EXPERIENCE
OUR NUMBERS SPEAK LOUDER
OUR NUMBERS SPEAK LOUDER
INDUSTRIES WE HAVE TRANSFORMED
MAKING INDIA PROUD
Clients admire us as
Thought Leaders
Technology Launch Pad
We're Fast.We're Agile.We're Outcome Focused
Exemplary Business Transformation
Our Customers love us because we nurture them

02
We helped them
protect their
business (IT Security)
For one of the largest MNC
dairies - 45%
reduction in their Security
Incident MTTR

03
We helped them
achieve faster than
ever (Automation)
For a TELECOM giant - 60%
reduction in
time to market with agile
process automation

04
We helped them
govern, manage risk
and stay complaint
(GRC)
For one of the fastest
growing DIGITAL AGENCIES -
75% reduction in data
collection time for ISO
compliance assessments

05
Digital Transformation
For one of the fastest
growing DIGITAL AGENCIES -
75% reduction in data
collection time for ISO
compliance assessments

02
We helped them protect their business (IT Security)
For one of the largest MNC dairies-45% reduction in their Security Incident MTTR

03
We helped them achieve faster than ever (Automation)
For a TELECOM giant -60% reduction in time to market with agiles process automation

04
We helped them govern, manage risk and stay complaint (GRC)
For one of the fastest growing DIGITAL AGENCIES - 75% reduction in data collection time for ISO compliance assessments

05
Digital Transformation
For one of the fastest growing DIGITAL AGENCIES - 75% reduction in data collection time for ISO compliance assessments
Our happy Customers
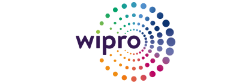
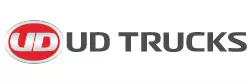
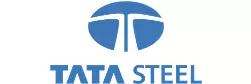
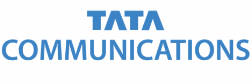
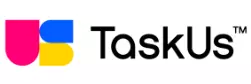
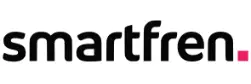
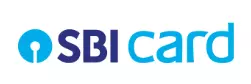
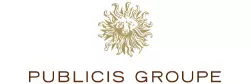
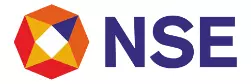
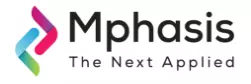
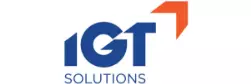
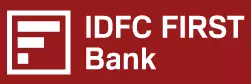
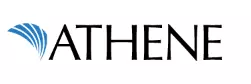
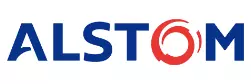
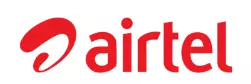
Customers Success
Case Studies
Useful links
Subscribe to our newsletter
Contact Us
India: Tower B, Floor S1 Urb Tech Trade Center Adjacent DPS School 35, Sector 132 Noida, Uttar Pradesh 201304
USA: One Boston Place, Suite 2600, Boston, MA 02108
UK: 806 Crown House, North Circular Road, North Circular Road, London, England, NW10 7PN
Singapore: 4, Shenton Way, Singapore 068807
© 2021 inMorphis Service Private Limited | Privacy Policy